Barrett Honors Thesis - Creative Project
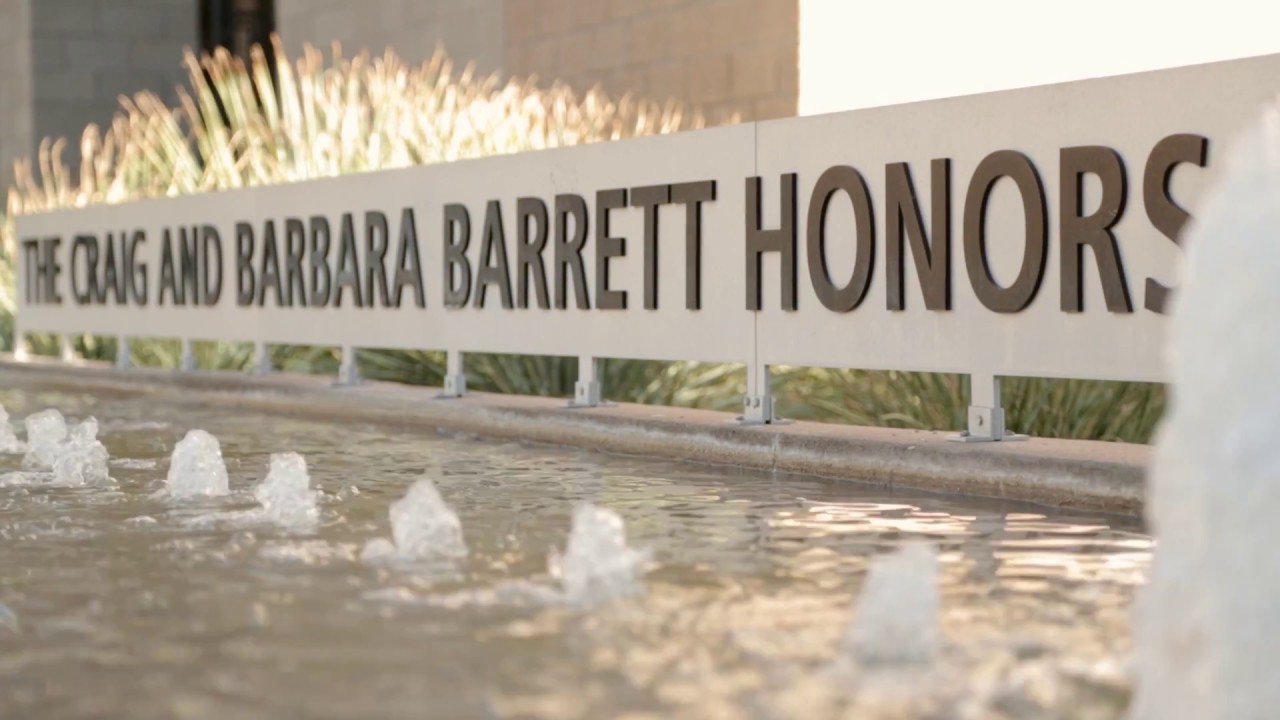
All Barrett students complete a thesis under the guidance of 2-3 committee members. For my thesis, I built a project of my own - iLieDown: Improved Display Orientation For Handheld Devices Using Convolutional Neural Networks.
Abstract
91% of smartphone and tablet users experience a problem with their device screen being oriented the wrong way during use [11]. In [11], the authors proposed iRotate, a previous solution which uses computer vision to solve the orientation problem. We propose iLieDown, an improved method of automatically rotating smartphones, tablets, and other device displays. This paper introduces a new algorithm to correctly orient the display relative to the user’s face using a convolutional neural network (CNN). The CNN model is trained to predict the rotation of faces in various environments through data augmentation, uses a confidence threshold, and analyzes multiple images to be accurate and robust. iLieDown is battery and CPU efficient, causes no noticeable lag to the user during use, and is 6x more accurate than iRotate.
Acknowledgements
Thanks to Professor Yezhou Yang and PhD student Zhiyuan Fang for their guidance while serving on my thesis committee.
What I've Learned
- Convolutional Neural Nets (CNNs) - I used a VGG style [13] CNN to create iLieDown.
- Image Data Augmentation - To train a robust CNN, one needs labeled image data. I learned how to do this with keras and I created images with varying brightness, rotation, and more.
- Swift and CoreML - I have deployed my solution to iOS 12.4 using Swift, which wasn't the easiest language to learn to be honest.
- Image pre-processing - Image pre-processing like laplacian filters and other edge detection algorithms makes it easier for the computer to perform image analysis and detect objects easier.